How much is your customer worth?
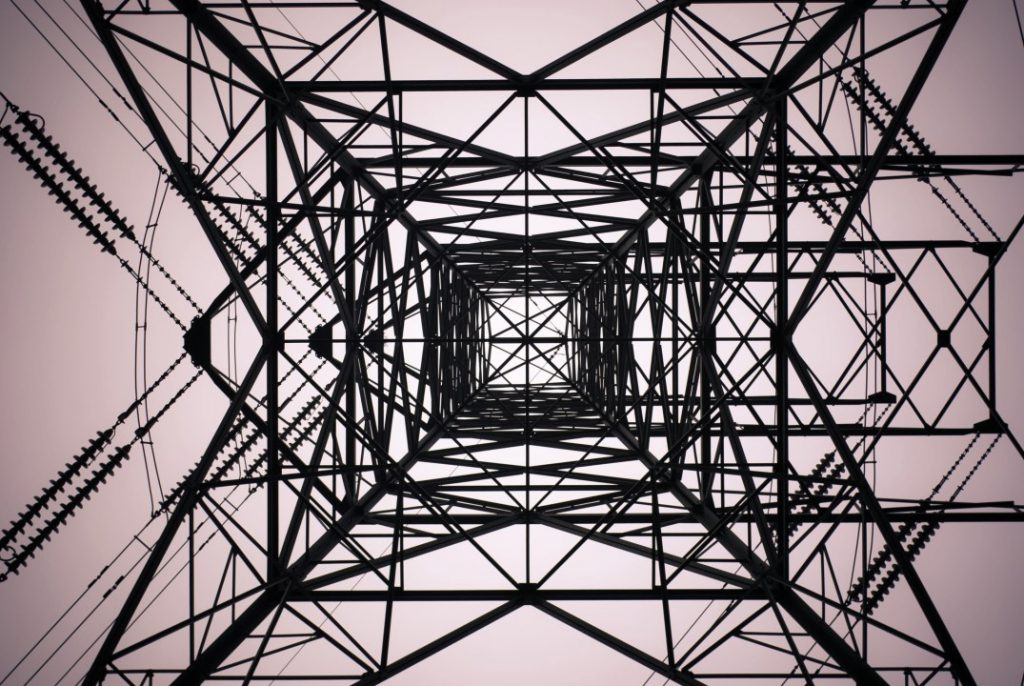
Our client is a multinational leader in the energy sector with investments in extraction, generation and distribution, with a significant presence in Europe and Latin America. It is currently developing business intelligence initiatives, exploiting its data with embedded solutions on cloud platforms.
The problem it had was big because, to generate any use case, it needed to consult countless sources of information generated manually by various departments, including text files and spreadsheets, but not just that, it also had to use information systems ranging from Oracle DB to Salesforce.
«The problem it had was big because, to generate any use case, it needed to consult countless sources of information generated manually»
The solution was clear; all the necessary information needed to be concentrated in a single, secure, continually available, organised and, above all, cost-efficient place. The decision was to implement a Data Lake in the AWS Cloud.
In project evolution, the client was concerned about the vulnerabilities of its local servers, where they have had some problems with service availability and even the a computer virus intrusion, for which /bluetab proposes to migrate the most critical processes completely to the cloud. These include a customer segmentation model, developed in R.
Segmenting the customer portfolio requires an ETL developed in Python using Amazon Redshift as DWH, where a Big Data EMR cluster is also run on demand with tasks developed in Scala to handle large volumes of transaction information generated on a daily basis. The process results that were previously hosted and exploited from a MicroStrategy server now were developed in reports and dashboards using Power BI.
«…the new architecture design and better management of cloud services in their daily use enabled us to optimise cloud billing, reducing OPEX by over 50%»
Not only did we manage to integrate a significant quantity of business information into a centralised, governed repository, but the new architecture design and better management of cloud services in their daily use enabled us to optimise cloud billing, reducing OPEX by over 50%. Additionally, this new model enables accelerated development of any initiative requiring use of this data, thereby reducing project cost.

Now our customer wants to test and leverage the tools we put into their hands to answer a more complex question: how much are my customers worth?
Its traditional segmentation model in the distribution business was based primarily on an analysis of payment history and turnover. In this way they predict the possibility of defaults in new services, and the potential value of the customer in billing terms. All of this, crossed with financial statement information, still formed a model with ample room for improvement.
«At /bluetab we have experience in development of analytical models that ensure efficient and measurable application of the most suitable algorithms for each problem and each data set»
At /bluetab we have experience in development of analytical models that ensure efficient and measurable application of the most suitable algorithms for each problem and each data set, but the market now provides solutions as very mature analytical models that, with minimum parametrisation, enable good results while drastically reducing development time. As such, we used a well-proven CLV (Customer Lifetime Value) model to help our client evaluate the potential life-cycle value of its customers.
We have incorporated variables in the new scenario such as After-sales service costs (such as recovery management, CC incident resolution costs, intermediary billing agent costs, etc.), and Provisioning logistics costs into the customers’ income and expense data, making it possible to include data on geographical positioning for distribution costs, market maturity in terms of market share, or crossing with information provided by different market sources. This means our client can make better estimates of the value of its current and potential customers, and perform modelling and forecasting of profitability for new markets or new services.
The potential benefit from application of the analytical models depends on less “sexy” aspects, such as consistent organisation and governance of the data in the back office, the quality of the data provisioning the model, implementation of the model following DevOps best practices and constant communication with the client to ensure business alignment and to be able to extract/visualise conclusions of value from the information obtained. And at /bluetab we believe this is only possible with expert technical knowledge and a deep commitment to understanding our clients’ businesses.
«The potential benefit from application of the analytical models is only possible with expert technical knowledge and a deep commitment to understanding our clients’ businesses»